Fei Shang
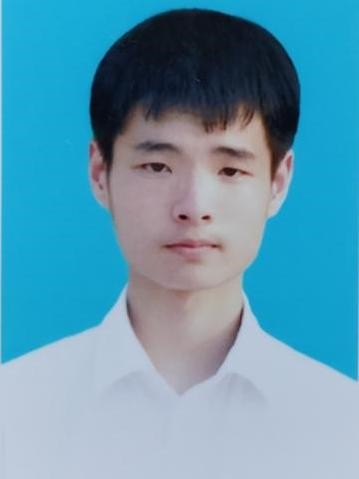
君子食无求饱,居无求安,敏于事而不讷于言,就有道而正焉
I am fortunate to be advised by Prof. Panlong Yang and Prof. Xiang-Yang Li at University of Science and Technology of China (USTC). My research interest is wireless sensing.
Awards
- Young Elite Scientists Sponsorship Program by CAST - Doctoral Student Special Plan (First session), 2024
- National Scholarship, 2022, 2024
- Intel Scholarship, 2023
- Outstanding Graduate Student of Anhui Province, 2025
Service
- Reviewer of IEEE IoTJ, IEEE TMC, IMWUT/Ubicomp, ACM TIoT
- The host at the BIGCOM Rising Star Forum, 2024
News
- [2025.04] 🎉🎉🎉 Our paper “The field-based model: a new perspective on RF-based material sensing” has been accepted by
SCIS
. - [2025.04] 🎉🎉🎉 Our paper “Measuring Discrete Sensing Capability for ISAC via Task Mutual Information” has been accepted by
SCIS
. - [2025.02] 🎉🎉🎉 Our paper “Pushing the Limits of WiFi-based Gait Recognition Towards Non-gait Human Behaviors” has been accepted by
IEEE TMC
.
Selected publications
- SCISThe Field-based Model: A New Perspective on RF-based Material SensingFei Shang, Haocheng Jiang, Panlong Yang, and 3 more authorsApr 2025
@misc{shang2024fieldbasedmodelnewperspective, title = {The Field-based Model: A New Perspective on RF-based Material Sensing}, author = {Shang, Fei and Jiang, Haocheng and Yang, Panlong and Yan, Dawei and Du, Haohua and Li, Xiang-Yang}, year = {2025}, month = apr, eprint = {2412.05640}, archiveprefix = {arXiv}, primaryclass = {eess.SP}, url = {https://arxiv.org/abs/2412.05640}, }
- SCISMeasuring discrete sensing capability for ISAC via task mutual informationFei Shang, Haohua Du, Panlong Yang, and 3 more authorsSCIENCE CHINA Information Sciences, Apr 2025
@article{shang2025measuringdiscretesensingcapability, title = {Measuring discrete sensing capability for ISAC via task mutual information}, author = {Shang, Fei and Du, Haohua and Yang, Panlong and He, Xin and Wang, Jingjing and Li, Xiang-Yang}, journal = {SCIENCE CHINA Information Sciences}, publisher = {Springer Science and Business Media LLC}, issn = {1869-1919}, year = {2025}, volume = {68}, number = {5}, month = apr, pages = {150308-}, url = {http://www.sciengine.com/publisher/Science China Press/journal/SCIENCE CHINA Information Sciences/68/5/10.1007/s11432-024-4374-y}, doi = {https://doi.org/10.1007/s11432-024-4374-y}, }
- IEEE TMCNon-Intrusive and Efficient Estimation of Antenna 3-D Orientation for WiFi APsDawei Yan, Panlong Yang, Fei Shang, and 2 more authorsIEEE Transactions on Mobile Computing, Mar 2025
The effectiveness of WiFi-based localization systems heavily relies on the spatial accuracy of WiFi AP. In real-world scenarios, factors such as AP rotation and irregular antenna tilt contribute significantly to inaccuracies, surpassing the impact of imprecise AP location and antenna separation. In this paper, we propose Anteumbler, a non-invasive, accurate, and efficient system for measuring the orientation of each antenna in physical space. By leveraging the fact that maximum received power occurs when a Tx-Rx antenna pair is perfectly aligned, we build a spatial angle model capable of determining antennas’ orientations without prior knowledge. However, achieving comprehensive coverage across the spatial angle necessitates extensive sampling points. To enhance efficiency, we exploit the orthogonality of antenna directivity and polarization, and adopt an iterative algorithm, thereby reducing the number of sampling points by several orders of magnitude. Additionally, to attain the required antenna orientation accuracy, we mitigate the influence of propagation distance using a dual plane intersection model while filtering out ambient noise. Our real-world experiments, covering six antenna types, two antenna layouts, two antenna separations (λ/2λ/2 and \lambdaλ ), and three AP heights, demonstrate that Anteumbler achieves median errors below \text6^\circ6∘ for both elevation and azimuth angles, and exhibits robustness in NLoS and dynamic environments. Moreover, when integrated into the reverse localization system, Anteumbler deployed over LocAP reduces antenna separation error by 10 \,\mathrmmm10 mm , while for user localization system, its integration over SpotFi reduces user localization error by more than 1 \,\mathrmm1m.
@article{yan2025anteumbler, author = {Yan, Dawei and Yang, Panlong and Shang, Fei and Freris, Nikolaos M. and Yan, Yubo}, journal = {IEEE Transactions on Mobile Computing}, title = {Non-Intrusive and Efficient Estimation of Antenna 3-D Orientation for WiFi APs}, year = {2025}, volume = {24}, number = {3}, pages = {1453-1468}, keywords = {Antenna measurements;Antennas;Location awareness;Wireless fidelity;Receiving antennas;Accuracy;Antenna arrays;Sensors;Manuals;Dipole antennas;Antenna orientation;polarization matching;WiFi localization}, doi = {10.1109/TMC.2024.3485228}, issn = {1558-0660}, month = mar }
- IEEE TMCPushing the Limits of WiFi-Based Gait Recognition Towards Non-Gait Human BehaviorsDawei Yan, Panlong Yang, Fei Shang, and 3 more authorsIEEE Transactions on Mobile Computing , Feb 2025
WiFi-based gait recognition technologies have seen significant advancements in recent years. However, most existing approaches rely on a critical assumption: users must walk continuously and maintain a consistent body posture. This poses a substantial challenge when users engage in non-periodic or discontinuous behaviors (e.g., stopping, starting, or turning mid-walk), which can disrupt the extraction of gait-related features and degrade recognition performance. To address this issue, we propose freeGait, a novel approach designed to mitigate the impact of non-gait behaviors in WiFi-based gait recognition systems. Our solution models this problem as domain adaptation, where we learn domain-independent representations to isolate gait features from behavior-dependent noise. We treat human behaviors with labeled user data as source domains and behaviors without user labels as target domains. However, applying domain adaptation directly is challenging due to the ambiguous classification boundaries in the target domains for WiFi signals. To overcome this, we align the posterior distributions between the source and target domains and constrain the conditional distribution within the target domains to enhance gait classification accuracy. Additionally, we implement a data augmentation module to generate data resembling the labeled data, while supervised learning ensures distinctiveness between users. Our experiments, conducted with 20 participants across 3 different scenarios, demonstrate that freeGait can accurately predict data across 15 domains by labeling only a small subset from 6 source domains, achieving up to a 45% improvement in user classification accuracy compared to existing methods.
@article{freegait-tmc, author = {Yan, Dawei and Yang, Panlong and Shang, Fei and Han, Feiyu and Yan, Yubo and Li, Xiang-Yang}, journal = { IEEE Transactions on Mobile Computing }, title = {{ Pushing the Limits of WiFi-Based Gait Recognition Towards Non-Gait Human Behaviors }}, year = {2025}, volume = {}, number = {01}, issn = {1558-0660}, pages = {1-17}, keywords = {Behavioral sciences;Legged locomotion;Gait recognition;Wireless fidelity;Feature extraction;Transceivers;Accuracy;Noise;Working environment noise;Sensors}, doi = {10.1109/TMC.2025.3540863}, url = {https://doi.ieeecomputersociety.org/10.1109/TMC.2025.3540863}, publisher = {IEEE Computer Society}, address = {Los Alamitos, CA, USA}, month = feb }
- JCRDSurvey on Low Power Sensing of AIoTXiangyang Li, Fei Shang, Yubo Yan, and 5 more authorsJisuanji Yanjiu yu Fazhan/Computer Research and Development, Nov 2024Artificial intelligence of thing;Digital analog drive;Internet of thing;Large-scales;Low-power consumption;Low-power sensing;Lower-power consumption;Mobile-computing;Sensing;Sensing modalities;
With the deepening integration of human-machine-object fusion, an increasing number of lightweight and large-scale sensing demands are emerging. To meet the deployment needs of multiple scenarios and large scales, low-cost and low-power sensing solutions are becoming increasingly favored. However, there are still some common and specific challenges in the field of low-power sensing that hinder their further development and practical application. Although many excellent reviews have been conducted on a specific sensing modality or application, there is still a lack of work that sorts out the entire field of low-power sensing. In this paper, we summarize recent low-power sensing, introduce three types of sensing modalities including inertial measurement unit (IMU), microphone, and radio frequency signals, summarize their related challenges, and introduce relevant solutions from hardware and software levels. Finally, we introduce the applications of sensing schemes in different scenarios from four aspects: surface sensing, property sensing, physiological sensing, and anti-sensing, along the direction from surface to inside, from object to human body, and from sensing to safety, and summarize several prospects for exploration.
@article{iotsurvey, language = {Chinese}, copyright = {Compendex}, title = {Survey on Low Power Sensing of AIoT}, journal = {Jisuanji Yanjiu yu Fazhan/Computer Research and Development}, author = {Li, Xiangyang and Shang, Fei and Yan, Yubo and Wang, Shanyue and Han, Feiyu and Chi, Guoxuan and Yang, Zheng and Chen, Xiaojiang}, volume = {61}, number = {11}, year = {2024}, pages = {2754 - 2775}, issn = {10001239}, note = {Artificial intelligence of thing;Digital analog drive;Internet of thing;Large-scales;Low-power consumption;Low-power sensing;Lower-power consumption;Mobile-computing;Sensing;Sensing modalities;}, url = {http://dx.doi.org/10.7544/issn1000-1239.202440396}, month = nov, doi = {10.7544/issn1000-1239.202440396} }
- SenSysPh.D. Forum: Field Sensing Model, A New Foundation for RF SensingFei ShangIn Proceedings of the 22nd ACM Conference on Embedded Networked Sensor Systems, Hangzhou, China, Nov 2024
In recent years, radio frequency (RF) signal-based sensing has garnered significant attention due to its ubiquity, with numerous applications emerging in areas such as target localization, material recognition, and health monitoring. However, current sensing models are often based on ray tracing, which, although computationally convenient, can become severely distorted when the target size is not much larger than the wavelength. Additionally, using signals with smaller wavelengths to mitigate this issue is not always feasible. Noting that RF signals are a form of electromagnetic waves, we have explored the development of field sensing models directly based on Maxwell’s equations. These models can finely characterize phenomena such as diffraction and multiple scattering, thereby enhancing the upper limits of sensing system capabilities. Based on this approach, we have achieved integrated material recognition and imaging of centimeter-scale targets using WiFi signals. This work has been accepted for presentation at Ubicomp 2024.
@inproceedings{Forum, author = {Shang, Fei}, title = {Ph.D. Forum: Field Sensing Model, A New Foundation for RF Sensing}, year = {2024}, isbn = {9798400706974}, publisher = {Association for Computing Machinery}, address = {New York, NY, USA}, url = {https://doi.org/10.1145/3666025.3699668}, doi = {10.1145/3666025.3699668}, booktitle = {Proceedings of the 22nd ACM Conference on Embedded Networked Sensor Systems}, pages = {920–922}, numpages = {3}, keywords = {wireless sensing, radio frequency, field model}, location = {Hangzhou, China}, series = {SenSys '24}, month = nov }
- UbicompEarSpeech: Exploring In-Ear Occlusion Effect on Earphones for Data-efficient Airborne Speech EnhancementFeiyu Han, Panlong Yang, You Zuo, and 3 more authorsProc. ACM Interact. Mob. Wearable Ubiquitous Technol., Sep 2024
Earphones have become a popular voice input and interaction device. However, airborne speech is susceptible to ambient noise, making it necessary to improve the quality and intelligibility of speech on earphones in noisy conditions. As the dual-microphone structure (i.e., outer and in-ear microphones) has been widely adopted in earphones (especially ANC earphones), we design EarSpeech which exploits in-ear acoustic sensory as the complementary modality to enable airborne speech enhancement. The key idea of EarSpeech is that in-ear speech is less sensitive to ambient noise and exhibits a correlation with airborne speech. However, due to the occlusion effect, in-ear speech has limited bandwidth, making it challenging to directly correlate with full-band airborne speech. Therefore, we exploit the occlusion effect to carry out theoretical modeling and quantitative analysis of this cross-channel correlation and study how to leverage such cross-channel correlation for speech enhancement. Specifically, we design a series of methodologies including data augmentation, deep learning-based fusion, and noise mixture scheme, to improve the generalization, effectiveness, and robustness of EarSpeech, respectively. Lastly, we conduct real-world experiments to evaluate the performance of our system. Specifically, EarSpeech achieves an average improvement ratio of 27.23% and 13.92% in terms of PESQ and STOI, respectively, and significantly improves SI-SDR by 8.91 dB. Benefiting from data augmentation, EarSpeech can achieve comparable performance with a small-scale dataset that is 40 times less than the original dataset. In addition, we validate the generalization of different users, speech content, and language types, respectively, as well as robustness in the real world via comprehensive experiments. The audio demo of EarSpeech is available on https://github.com/EarSpeech/earspeech.github.io/.
@article{EarSpeech, author = {Han, Feiyu and Yang, Panlong and Zuo, You and Shang, Fei and Xu, Fenglei and Li, Xiang-Yang}, title = {EarSpeech: Exploring In-Ear Occlusion Effect on Earphones for Data-efficient Airborne Speech Enhancement}, year = {2024}, issue_date = {September 2024}, publisher = {Association for Computing Machinery}, address = {New York, NY, USA}, volume = {8}, number = {3}, url = {https://doi.org/10.1145/3678594}, doi = {10.1145/3678594}, journal = {Proc. ACM Interact. Mob. Wearable Ubiquitous Technol.}, month = sep, articleno = {104}, numpages = {30}, keywords = {Earphone-based Sensing and Computing, In-ear Acoustic Sensing, Occlusion Effect, Speech Enhancement} }
- MobiHocfreeGait: Liberalizing Wireless-based Gait Recognition to Mitigate Non-gait Human BehaviorsDawei Yan, Panlong Yang, Fei Shang, and 3 more authorsIn Proceedings of the Twenty-Fifth International Symposium on Theory, Algorithmic Foundations, and Protocol Design for Mobile Networks and Mobile Computing, Athens, Greece, Jun 2024
Recently, WiFi-based gait recognition technologies have been widely studied. However, most of them work on a strong assumption that users need to walk continuously and periodically under a constant body posture. Thus, a significant challenge arises when users engage in non-periodic or discontinuous behaviors (e.g., stopping and going, turning around during walking). This is because variations of non-gait behaviors interfere with the extraction of gait-related features, resulting in recognition performance degradation. To solve this problem, we propose freeGait, which aims to mitigate the user’s non-gait behaviors of WiFi-based gait recognition system. Specifically, we model this problem as domain adaptation, by learning domain-independent representations to extract behavior-independent gait features. We consider human behaviors with labels of users as source domains, and human behaviors without labels of users as target domains. However, directly applying domain adaptation to our specific problem is challenging, because the classification boundaries of the unknown target domains are unclear for WiFi signals. We align the posterior distributions of the source and target domains, and constrain the conditional distribution of the target domains to optimize the gait classification accuracy. To obtain enough source domains data, we build a data augmentation module to generate data similar to the labeled data, and use supervised learning to make the data different between users. We conduct experiments with 20 people and 3 different scenarios, and the results show that accurate predictions of a total of 15 domains data can be achieved by only collecting and labeling a small amount of data from 6 source domains, and user classification accuracy can be improved by up to 45% compared to other existing techniques.
@inproceedings{freeGait, author = {Yan, Dawei and Yang, Panlong and Shang, Fei and Han, Feiyu and Yan, Yubo and Li, Xiang-Yang}, title = {freeGait: Liberalizing Wireless-based Gait Recognition to Mitigate Non-gait Human Behaviors}, year = {2024}, isbn = {9798400705212}, publisher = {Association for Computing Machinery}, address = {New York, NY, USA}, url = {https://doi.org/10.1145/3641512.3686362}, doi = {10.1145/3641512.3686362}, booktitle = {Proceedings of the Twenty-Fifth International Symposium on Theory, Algorithmic Foundations, and Protocol Design for Mobile Networks and Mobile Computing}, pages = {241–250}, numpages = {10}, keywords = {wifi-based sensing, gait recognition, domain adaptation, data augmentation}, location = {Athens, Greece}, series = {MobiHoc '24}, month = jun }
- IEEE TMCContactless and Fine-Grained Liquid Identification Utilizing Sub-6 GHz SignalsFei Shang, Panlong Yang, Yubo Yan, and 1 more authorIEEE Transactions on Mobile Computing, May 2024
The existing RF-based liquid identification systems usually rely on prior knowledge, such as pre-build database or the material and width information of the vessel. Furthermore, existing methods may not work in scenarios where the height of liquid is smaller than that of antenna. In this paper, we proposes LiqRay^++, a contactless system which can identify liquids in a fine-grained level without prior knowledge. To remove the effect of vessel, we build a dual-antenna model and craft a relative frequency response factor, exploring diversity of the permittivity in frequency domain. To eliminate the effect of different height, we devise the electric field distribution model at the receiving antenna, solving the unknown heights via spatio-differential model. Among eight different solvents, LiqRay^++ can identify alcohol solutions with a concentration difference of 1% with 92.9% accuracy. Even if the liquid height is about 4 cm, which is fairly lower than that of most antennas’ heights, the accuracy is more than 85%.
@article{LiqRay-TMC, author = {Shang, Fei and Yang, Panlong and Yan, Yubo and Li, Xiang-Yang}, journal = {IEEE Transactions on Mobile Computing}, title = {Contactless and Fine-Grained Liquid Identification Utilizing Sub-6 GHz Signals}, year = {2024}, volume = {23}, number = {5}, pages = {4992-5008}, keywords = {Liquids;Transmitting antennas;Attenuation;Antennas;Receiving antennas;Electric fields;Electromagnetic scattering;Contactless;fine-grained;liquid identification;wireless sensing}, doi = {10.1109/TMC.2023.3300356}, issn = {1558-0660}, month = may }
- UbicompLiquImager: Fine-grained Liquid Identification and Container Imaging System with COTS WiFi DevicesFei Shang, Panlong Yang, Dawei Yan, and 2 more authorsProc. ACM Interact. Mob. Wearable Ubiquitous Technol., Mar 2024
WiFi has gradually developed into one of the main candidate technologies for ubiquitous sensing. Based on commercial off-the-shelf (COTS) WiFi devices, this paper proposes LiquImager, which can simultaneously identify liquid and image container regardless of container shape and position. Since the container size is close to the wavelength, diffraction makes the effect of the liquid on the signal difficult to approximate with a simple geometric model (such as ray tracking). Based on Maxwell’s equations, we construct an electric field scattering sensing model. Using few measurements provided by COTS WiFi devices, we solve the scattering model to obtain the medium distribution of the sensing domain, which is used for identifing and imaging liquids. To suppress the signal noise, we propose LiqU-Net for image enhancement. For the centimeter-scale container that is randomly placed in an area of 25 cm \texttimes 25 cm, LiquImager can identify the liquid more than 90% accuracy. In terms of container imaging, LiquImager can accurately find the edge of the container for 4 types of containers with a volume less than 500 ml.
@article{LiquImager, author = {Shang, Fei and Yang, Panlong and Yan, Dawei and Zhang, Sijia and Li, Xiang-Yang}, title = {LiquImager: Fine-grained Liquid Identification and Container Imaging System with COTS WiFi Devices}, year = {2024}, issue_date = {March 2024}, publisher = {Association for Computing Machinery}, address = {New York, NY, USA}, volume = {8}, number = {1}, url = {https://doi.org/10.1145/3643509}, doi = {10.1145/3643509}, journal = {Proc. ACM Interact. Mob. Wearable Ubiquitous Technol.}, month = mar, articleno = {15}, numpages = {29}, keywords = {Complex permittivity, Electromagnetic waves, Liquid identification, Wireless sensing} }
- UbicompWi-Painter: Fine-grained Material Identification and Image Delineation Using COTS WiFi DevicesDawei Yan, Panlong Yang, Fei Shang, and 2 more authorsProc. ACM Interact. Mob. Wearable Ubiquitous Technol., Jan 2024
WiFi has gradually developed into one of the main candidate technologies for indoor environment sensing. In this paper, we are interested in using COTS WiFi devices to identify material details, including location, material type, and shape, of stationary objects in the surrounding environment, which may open up new opportunities for many applications. Specifically, we present Wi-Painter, a model-driven system that can accurately detects smooth-surfaced material types and their edges using COTS WiFi devices without modification. Different from previous arts for material identification, Wi-Painter subdivides the target into individual 2D pixels, and simultaneously forms a 2D image based on identifying the material type of each pixel. The key idea of Wi-Painter is to exploit the complex permittivity of the object surface which can be estimated by the different reflectivity of signals with different polarization directions. In particular, we construct the multi-incident angle model to characterize the material, using only the power ratios of the vertically and horizontally polarized signals measured at several different incident angles, which avoids the use of inaccurate WiFi signal phases. We implement and evaluate Wi-Painter in the real world, showing an average classification accuracy of 93.4% for different material types including metal, wood, rubber and plastic of different sizes and thicknesses, and across different environments. In addition, Wi-Painter can accurately detect the material type and edge of the word "LOVE" spliced with different materials, with an average size of 60cm \texttimes 80cm, and material edges with different orientations.
@article{Wi-Painter, author = {Yan, Dawei and Yang, Panlong and Shang, Fei and Jiang, Weiwei and Li, Xiang-Yang}, title = {Wi-Painter: Fine-grained Material Identification and Image Delineation Using COTS WiFi Devices}, year = {2024}, issue_date = {December 2023}, publisher = {Association for Computing Machinery}, address = {New York, NY, USA}, volume = {7}, number = {4}, url = {https://doi.org/10.1145/3633809}, doi = {10.1145/3633809}, journal = {Proc. ACM Interact. Mob. Wearable Ubiquitous Technol.}, month = jan, articleno = {203}, numpages = {25}, keywords = {Complex permittivity, Material identification, Object imaging, Wireless sensing} }
- ACM TOSNTamera: Contactless Commodity Tracking, Material and Shopping Behavior Recognition Using COTS RFIDsFei Shang, Panlong Yang, Jie Xiong, and 2 more authorsACM Trans. Sen. Netw., Feb 2023
RFID technology has recently been exploited for not only identification but also fine-grained trajectory tracking and gesture recognition. While contact-based (a tag is attached to the target of interest) sensing has achieved promising results, contactless sensing still faces severe challenges such as low accuracy and inability to sense multiple targets simultaneously in proximity, restricting its applicability in real-world deployment. In this work, we present Tamera, a contactless RFID-based sensing system, which significantly improves the tracking accuracy, enables multi-commodity tracking, and even material and shopping behavior recognition. We successfully address multiple technical challenges, and design and implement our prototype on commodity RFID devices. We test the positioning accuracy of Tamera in a 5 m \texttimes 6 m laboratory. Tamera achieves a median error of 1.3 cm and 2.7 cm for contactless single- and multi-commodity tracking, respectively. In our laboratory, two shelves commonly found in the supermarket are arranged and the goods are placed on them. Tamera successfully localizes and identifies the material type (metal, plastic, paper, and glass) of the commodities on the shelf with an accuracy higher than 95%. Tamera successfully recognizes four shopping behaviors (taking commodity, replacing commodity, buying commodity, and invoking commodity) with an accuracy higher than 93%.
@article{Tamera, author = {Shang, Fei and Yang, Panlong and Xiong, Jie and Feng, Yuanhao and Li, Xiangyang}, title = {Tamera: Contactless Commodity Tracking, Material and Shopping Behavior Recognition Using COTS RFIDs}, year = {2023}, issue_date = {May 2023}, publisher = {Association for Computing Machinery}, address = {New York, NY, USA}, volume = {19}, number = {2}, issn = {1550-4859}, url = {https://doi.org/10.1145/3563777}, doi = {10.1145/3563777}, journal = {ACM Trans. Sen. Netw.}, month = feb, articleno = {43}, numpages = {24}, keywords = {shopping behavior, material, sensing, tracking, contactless, RFID} }
- UbicompPackquID: In-packet Liquid Identification Using RF SignalsFei Shang, Panlong Yang, Yubo Yan, and 1 more authorProc. ACM Interact. Mob. Wearable Ubiquitous Technol., Jan 2023
There are many scenarios where the liquid is occluded by other items (e.g. books in a packet), in which existing RF-based liquid identification methods are generally not suitable. Moreover, status methods are not applicable when the height of the liquid to be tested changes. This paper proposes PackquID, an RF-based in-packet liquid identification system, which can identify liquid without prior knowledge. In dealing with the obstruction of other items and the unknown container, we utilize a dual-antenna model and craft a relative frequency response factor, exploring the diversity of the permittivity in the frequency domain. In tackling the variable liquid height, we extend our model to 3D scope by analyzing the electric field distribution and solving the height effect via spatial-differential model. With 500 pages of printer paper obscured, PackquID can identify 9 common liquids, including Coca-Cola and Pepsi, with an accuracy of over 86% for 4 different packets (canvas bag, paper bag, backpack, and box) and 4 different containers. Nevertheless, PackquID can still identify liquids with an accuracy rate of over 87%, even when the liquid height changes from 4 cm to 12 cm.
@article{PackquID, author = {Shang, Fei and Yang, Panlong and Yan, Yubo and Li, Xiang-Yang}, title = {PackquID: In-packet Liquid Identification Using RF Signals}, year = {2023}, issue_date = {December 2022}, publisher = {Association for Computing Machinery}, address = {New York, NY, USA}, volume = {6}, number = {4}, url = {https://doi.org/10.1145/3569469}, doi = {10.1145/3569469}, journal = {Proc. ACM Interact. Mob. Wearable Ubiquitous Technol.}, month = jan, articleno = {181}, numpages = {27}, keywords = {Complex permittivity, Electromagnetic waves, Liquid identification, Wireless sensing} }
- MobiComLiqRay: non-invasive and fine-grained liquid recognition systemFei Shang, Panlong Yang, Yubo Yan, and 1 more authorIn Proceedings of the 28th Annual International Conference on Mobile Computing And Networking, Sydney, NSW, Australia, Oct 2022
The existing RF-based liquid identification methods commonly require a training network of liquid or the container information, such as material and width. Moreover, status quo methods are inapplicable when the solution height is lower than that of the antenna, which is generally unknown either. This paper proposes LiqRay, an RF-based solution, retaining non-invasive and fine-grained liquid recognition abilities, thus can recognize unknown solutions without prior knowledge. In dealing with the unknown container material and width, we utilize a dual-antenna model and craft a relative frequency response factor, exploring diversity of the permittivity in frequency domain. In tackling the unknown heights of solution and antenna, we devise the electric field distribution model at the receiving antenna, solving the unknown heights via spatio-differential model. Among eight different solvents, LiqRay can identify alcohol solutions with a concentration difference of 1% with 94.92% accuracy. Nevertheless, LiqRay can obtain the relative frequency response factor with a relative error of 6.7% without being affected by the height of the solution. Even if it is merely 4 cm, this is fairly lower than that of most antennas’ heights, since the operating frequency is around 2 GHz.
@inproceedings{liqray, author = {Shang, Fei and Yang, Panlong and Yan, Yubo and Li, Xiang-Yang}, title = {LiqRay: non-invasive and fine-grained liquid recognition system}, year = {2022}, month = oct, isbn = {9781450391818}, publisher = {Association for Computing Machinery}, address = {New York, NY, USA}, url = {https://doi.org/10.1145/3495243.3560540}, doi = {10.1145/3495243.3560540}, booktitle = {Proceedings of the 28th Annual International Conference on Mobile Computing And Networking}, pages = {296–309}, numpages = {14}, keywords = {wireless sensing, material identification, liquid identification, contactless sensing, complex permittivity}, location = {Sydney, NSW, Australia}, series = {MobiCom '22}, }